Artificial Intelligence and Machine Learning :-
- roshnipatil1314
- Oct 19, 2020
- 5 min read
What is machine learning and artificial intelligence ?
Machine learning :-
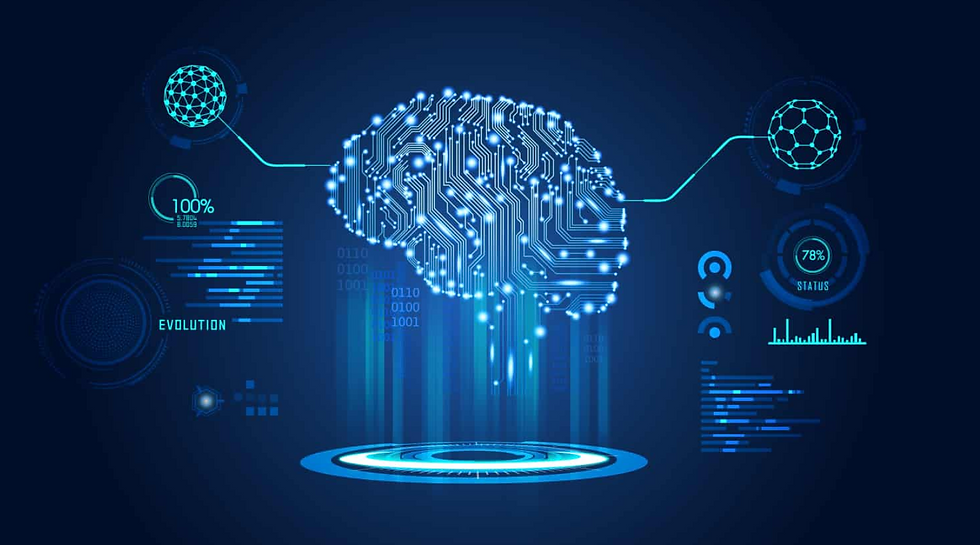
Machine learning basically builds the algorithms with the help of the data provide to it , so that they can done the certain tasks. It is the subset of the artificial intelligence . In simple sentence we can say that it's main function is collect the data, experienced it and predict the result . It is totally focused on predictions . It's algorithm is used in a wide variety of applications that provides systems the ability to automatically learn and improve from experience without being explicitly programmed.
Artificial Intelligence :-

The term artificial intelligence (AI) refers to any human-like intelligence exhibited by a computer, robot, or other machine. In popular usage, artificial intelligence refers to the ability of a computer or machine to mimic the capabilities of the human mind—learning from examples and experience, recognizing objects, understanding and responding to language, making decisions, solving problems—and combining these and other capabilities to perform functions a human might perform, such as greeting a hotel guest or driving a car.
Use Cases of ML :-
Image & Video Recognition:-
Over the past few years, image and video recognition have experienced rapid progress due to advances in deep learning (DL), which is a subset of machine learning.Image and video recognition are used for face recognition, object detection, text detection (printed and handwritten), logo and landmark detection, visual search, reverse image search, image composition, and image curation.
Speech Recognition:-
Speech recognition is another area of machine learning that allows machines to “mimic” humans due to AI, ML, and deep learning techniques. In this case, however, not image pixels, or frame-by-frame videos, but audio files get analyzed and processed by neural networks to translate audio into a text file.
Fraud Detection:-
According to The Nilson Report’s 2016 study, online credit card fraud was expected to cost banks and financial institutions $32bn in 2020. Unfortunately to the bad actors, ML’s fraud detection capabilities weren’t factored into the results.
In machine learning, fraud detection belongs to a separate class of classification problems, along with spam detection, recommendation systems, and loan default prediction.
Patient Diagnosis:-
Machine learning is extensively used in healthcare, offering doctors and health professionals tools to efficiently collect and analyze patients’ data for better diagnosis.
Efficient patient diagnosis is enabled by data, which comes in many shapes and sizes: MRIs, CAT scans, physician notes, pathology reports, bedside monitors, and more.
Anomaly Detection:-
Anomaly detection is widely used in manufacturing to increase productivity and efficiency, reduce costs, and optimize downtime.
Here’s how anomaly detection works:
Sensors are installed onto a piece of equipment to collect data
ML models process the data to find anomalous data
Anomalous data is analyzed to identify a specific problem pertaining to it
The problem is preemptively resolved to avoid equipment failure
Inventory Optimization:-
Inventory optimization is one of the most unnoticed, yet crucially important use cases of machine learning. It enables the machines to control how much stock to keep and how to keep it in the warehouse in the most efficient manner, to ensure that the supply chain won’t run dry.
Demand Forecasting :-
Demand forecasting is applied in a wide variety of industries, from e-commerce and retail to transportation and manufacturing. It feeds historical data to ML models to predict the amount of something — be it products, services, raw material, power, or anything else — to be consumed on the market in a given period of time.
It allows businesses to collect and process data from the entire supply chain, which increases efficiency and reduces overheads.
Recommendation Systems:-
Recommendation or recommender systems are one of the most ubiquitous applications of machine learning in daily life. These systems are used in search engines, e-commerce websites (e.g. Amazon, eBay), entertainment platforms (e.g. Netflix, Google Play), games, and multiple Web & mobile apps.
Intrusion Detection:-
ML-powered intrusion detection is the lifeblood of adaptive intrusion detection systems (IDS), which monitor networks in real time to identify and cope with malicious traffic or intrusion techniques, like brute force, infiltration, and unauthorized access.
Case Study :- How Netflix uses AI & ML
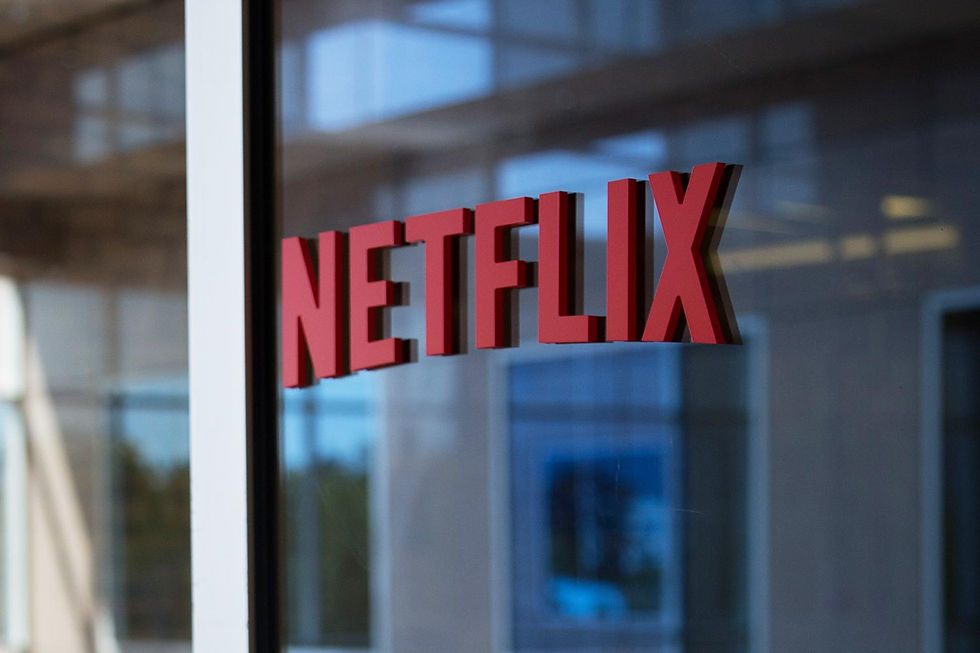
As the world’s leading Internet television network with over 160 million members in over 190 countries, Netflix members enjoy hundreds of millions of hours of content per day, including original series, documentaries and feature films. They invest heavily in machine learning to continually improve their member experience and optimize it's service end-to-end.
Historically, Netflix trusted heavily on the ratings given by customers for videos when shipping DVDs by mail. But now Netflix has access to a much large set of data which each member watches, when they watch, the place on the Netflix screen the customer found the video, recommendations the customer didn't choose, and the popularity of videos in the catalog. All the data gets fed into many algorithms power-driven by statistical and machine learning techniques. This approach uses both approaches like supervised and unsupervised. Supervised approach includes classification and regression while unsupervised approach includes reduction through clustering or compression.
Machine learning impacts many exciting areas throughout their company. They are also using machine learning to help shape their catalog of movies and TV shows by learning characteristics that make content successful. They use it to optimize the production of original movies and TV shows in Netflix’s rapidly growing studio. It also powers their advertising spend, channel mix, and advertising creative so that they can find new members who will enjoy Netflix.
As we all know most Netflix recommendation engines are powered by machine learning algorithms. Traditionally, they collect batches of data on how their members use the service. Then they run a new machine learning algorithm on this batch of data. Next, they test this new algorithm by testing A / B against the current production system.

🎯 Personalization of Movie Recommendations
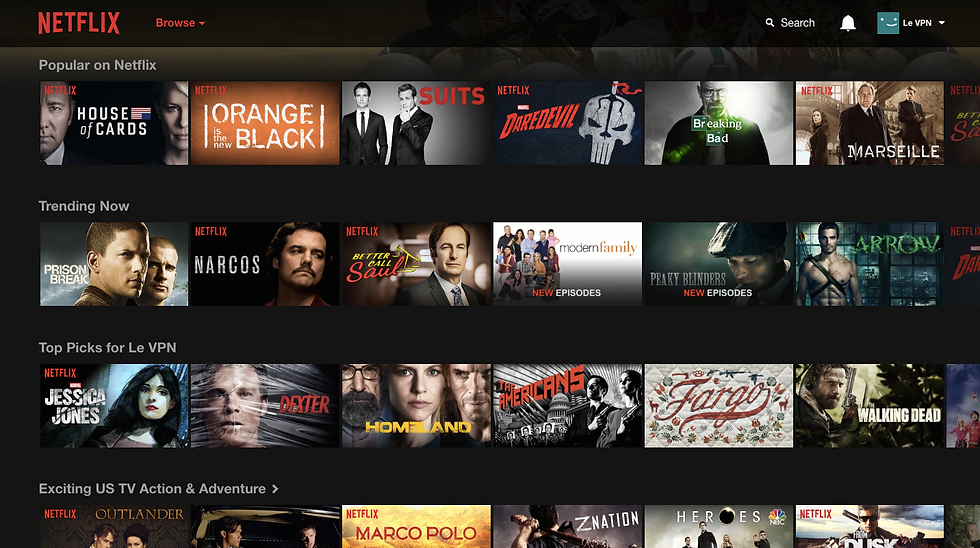
Users who watch A are likely to watch B. This is perhaps the most well known feature of a Netflix. Netflix uses the watching history of other users with similar tastes to recommend what you may be most interested in watching next so that you stay engaged and continue your monthly subscription for more.
🎯 Personalized Artwork
Every movie recommended by Netflix comes with associated Artwork. The Artwork that comes along with a movie suggestion is not common for everyone. Like movie recommendation, the Artwork related to a show is also personalized. All the members do not see a single best Artwork. A portfolio of Artwork will be created for a specific title. Depending on the taste and preference of the audience machine learning algorithm will choose an artwork which maximizes the chances of viewing the title.
A portfolio of Artwork created for the title ‘Stranger Things’:-
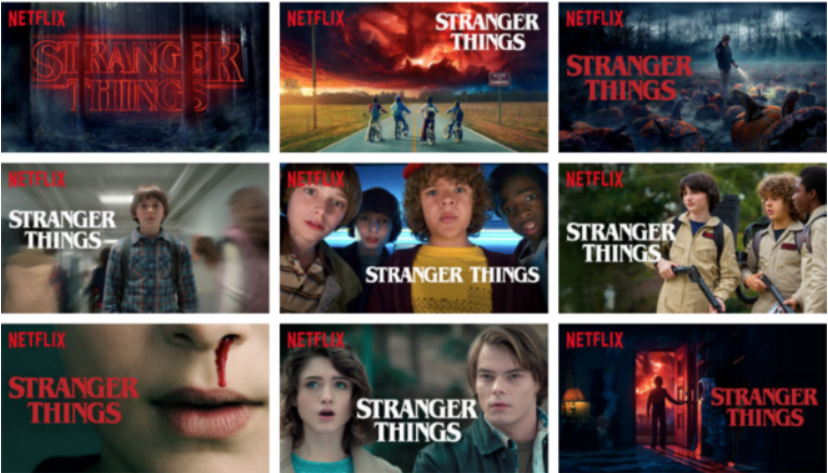
Comments